How to Analyze Data: A Step-by-Step Guide
Data analysis is a cornerstone for businesses, shaping their strategic decisions and future planning. And if you’ve ever felt a sense of dread when it’s time to analyze the performance of your latest marketing campaign or the results of your current sales iniative, then you’re not alone.
Source: WebFX
The ability to effectively analyze data can lead to groundbreaking insights, fostering growth and giving companies a competitive advantage. Sometimes, however, it’s easier said than done. Organizations are often faced with an overwhelming amount of data, making it difficult and time-consuming to collect, process and analyze efficiently. The time it takes to compile the data can be substantial, especially when most of the time, it is stored in a variety of platforms and systems. The real challenge of analyzing data effectively lies in transforming this vast array of data into actionable insights. But before we give you our top tips on how to analyze your data effectively, let’s dive a little deeper into data analysis, what it is and why it’s so important.
Source: Gartner
What is data analysis?
Data analysis is the process of examining, cleaning, transforming, and interpreting data to discover useful information, inform conclusions, and support decision-making. Think of it as piecing together a puzzle of your business data. It’s all about sifting through heaps of information, organizing it and then making sense of what it actually tells you. Why does this matter? Well, it's the difference between guessing and making decisions that are backed up by real data. It's not just number-crunching; it's gaining insights about your business, your customers, and using this information to enhance your business.
Why is data analysis important?
Data analysis is a vital part of any modern business strategy as it provides a scientific basis for decisions, reducing the reliance on intuition or unreliable anecdotal evidence. Here are just a few concrete reasons why data analysis is crucial for business success:
- Improved customer experience: By understanding customer behaviours and needs through data, businesses can offer better, more personalized support.
Example: If the data from an online retailer shows a customer frequently buys sports equipment, the store can personalize their emails to highlight new sports-related products, sales in that product category, or even include more sports-related content. This not only makes the shopping experience more relevant and engaging for the customer but also increases the likelihood of repeat purchases and customer loyalty.
Source: Hubspot
- Better decision-making: Data analysis provides a comprehensive snapshot of the business, enhancing decision-making with insights into what's working, potential risks, and areas for improvement.
Example: A business might analyze social media engagement rates to help identify its most popular products or services. This information can guide their marketing strategy, leading to targeted promotions on those specific high-demand items.
Source: IBM
- Understanding customer behaviour: Analyzing data helps predict customer behaviours, allowing businesses to respond or adapt quickly to their needs.
Example: A company might analyze purchasing data to identify which products are frequently bought together. This analysis can reveal patterns in customer preferences and buying habits. The company can then create targeted marketing campaigns, bundle those specific products together for promotions, or even adjust their product development strategy to reflect these observed preferences.
- Competitor analysis: It provides valuable insights into competitors, helping businesses understand their strategies and identify opportunities for their own competitive advantages.
Example: A business might analyze the social media activity, customer reviews, and pricing strategies of its competitors. This analysis can reveal which marketing channels are most effective for their competitors, what customers like or dislike about their products, and how their pricing strategy affects market share. Armed with this information, the business can adjust its strategies to capitalize on competitors' weaknesses and emulate successful tactics, leading to a more competitive position.
How to analyze your data
Now that you have a better understanding of what data analysis is and why it’s important, let's jump into how to analyze your data.
1. Define your goals
Before analyzing your data, you need to carefully consider what you want to achieve or what exactly it is you’re looking for. This step is key as it steers you towards gathering the right data and choosing the best tools and methods. If you don’t do this, then you are probably going to waste time and resources collecting and looking at data that isn’t relevant or helpful to you.
Say you're a digital marketer and you notice a decline in engagement on your social media campaigns. Your primary goals might be:
- Uncovering the reasons for the decline
- Suggesting new or alternative strategies to boost engagement and reach
With these as your goals, your data analysis might explore:
- Any emerging trends in engagement metrics in recent campaigns
- Comparison of these trends with previous successful campaigns
- Shifts in audience interaction or content preferences
By focusing your analysis on these specific areas, you can collect relevant data, conduct meaningful analysis, and devise effective strategies to enhance your social media presence.
Once you've set your goals, it's important to figure out how you're going to track your progress. This means picking the right metrics and key performance indicators (KPIs) that align with your objectives. For instance, if boosting sales is your target, you should keep a close eye on metrics like total revenue, the number of transactions, or the average purchase value. Alternatively, if you're aiming to improve customer service efficiency, you might track metrics related to the performance of support staff and customer satisfaction levels.
2. Collecting your data
This next step is to collect your data from all relevant sources, relevant to your set goals. This can range from internal systems, like your CRM and email marketing software, to external sources such as market research or social media analytics. For most businesses, it’s beneficial to collect both quantitative and qualitative data. Quantitative data, which is numerical and can be measured, provides objective metrics like sales figures or website traffic stats.
Qualitative data, on the other hand, is descriptive and subjective, offering deeper insights into areas like customer sentiment, preferences, and motivations, and is usually obtained through surveys, interviews, or social media analysis. Combining both of these data types provides a more holistic view of your business landscape and allows for a richer analysis, helping to understand not just the 'what' but also the 'why' of your data.
A major hurdle in the data collection process is ensuring data quality. One common challenge is dealing with inconsistent data formats, especially when amalgamating data from various sources. These inconsistencies can skew analysis and lead to incorrect conclusions if not properly addressed. Another issue is missing values, which can arise from incomplete data collection or transfer errors. Missing values can significantly impact the reliability of your analysis, as they can lead to biased results or misinterpretations.
3. Cleaning your data
After you’ve gathered your data, you need to dedicate time to cleaning it to ensure you’re basing your decisions on the right information. This can include the following:
- Correct errors: Look for inaccuracies or inconsistencies in the data and correct them. This could involve fixing typos or standardizing data formats (like date, time, currency or percentages)
- Removing duplicates: Duplicates can skew results so be extra cautious when copying and pasting your data onto a spreadsheet manually.
- Filter irrelevant data: Consider deleting unnecessary rows or columns that aren’t adding any value or are not relevant to your goals.
- Handle missing values: Identify and address any missing information, either by filling in gaps or removing incomplete records.
Remember, the strength of your data analysis is directly tied to the quality and reliability of your data.
Source: Gartner
4. Analyze your data
After setting your goals and prepping your data, it's time to dive into the actual analysis. This is where you get to play detective with your data, using different methods to uncover hidden insights. Here are a few of the most common methods of data analysis:
- Text analysis: Text analysis, also known as text mining, is the process of extracting valuable information and insights from textual data. It involves analyzing text to understand the meaning, sentiment, or patterns. This technique can be used to extract meaningful information like understanding customer feedback, social media comments, or product reviews. For example, a company might use text analysis to sort through thousands of customer reviews to gauge overall sentiment about their product or service. This helps in identifying trends, customer needs, and potential areas for improvement.
- Statistical analysis: This uses mathematical and statistical techniques to interpret data and identify trends, patterns, and relationships. It's key in making informed decisions based on empirical evidence and is commonly used for tasks like market research, quality control, and forecasting future trends. For example, a company might analyze sales data to identify which products are the most popular or to understand how different factors like marketing spend influence sales. This analysis helps in making data-driven decisions, reducing uncertainty, and optimizing business strategies.
- Diagnostic analysis: This is a deeper dive into the 'why' of things that happen. This type of analysis refers to the process of examining data to understand the causes and reasons behind specific trends, patterns, or anomalies. If a company notices a sudden drop in website traffic or sales, diagnostic analysis can be used to investigate the underlying reasons. This might involve looking at changes in marketing strategies, examining external market conditions, or exploring changes in customer behaviour. The goal is to pinpoint the factors contributing to a particular outcome, which can then inform more effective strategies and solutions.
- Predictive analysis: Predictive analysis uses historical data, statistical algorithms, and machine learning techniques to predict future outcomes. It involves analyzing past trends and patterns to forecast future events, helping businesses anticipate market changes, customer behaviours, or potential risks. A retailer might use predictive analysis to forecast sales trends, optimize inventory levels, or tailor marketing campaigns to specific customer segments. This type of analysis is key for proactive decision-making and strategic planning, allowing businesses to stay ahead of the curve.
- Prescriptive analysis: Prescriptive analytics goes beyond predicting future trends by recommending specific actions based on the data analysis. It combines insights from various forms of analytics (descriptive, diagnostic, predictive) and applies them to decision-making. For example, prescriptive analytics might analyze market trends, customer data, and internal processes to not only predict future outcomes but also suggest the best course of action. This could involve optimizing supply chain operations, personalizing marketing strategies, or enhancing customer experiences. The key advantage of prescriptive analytics is its ability to provide actionable recommendations, helping businesses make more informed, data-driven decisions.
Top tips for analyzing your data
Interpreting results effectively is a crucial step in the data analysis process. It involves understanding the significance of your findings and how they align with your initial objectives. This phase requires a critical eye to discern patterns, anomalies, or correlations in your data. It's important to consider the context of the data and any external factors that might influence the results. Effective interpretation often involves looking beyond the numbers to understand the story they tell about your business, market trends, or customer behaviour.
Here are our top tips for analysing your data most effectively:
1. Data visualization
After you have analyzed your data, it's vital to understand how to bring these analyses to life. This is where data visualization comes into play. Data visualization is not just about making charts and graphs; it's about transforming data into a narrative that anyone can understand. It converts complex data into a visual story, which aids in decision-making, opportunity identification, and comprehending data significance.
Say you have a set of sales figures for the year. Without visualization, these figures are just a collection of numbers across rows on a spreadsheet. But, when displayed more visually (i.e. a bar chart or line graph) these figures become a clear, engaging story - showing trends, peaks, and troughs in your business cycle. Hurree is an excellent tool for this, making data visualization both straightforward and impactful, enabling you to quickly turn complex data sets into visually compelling stories, essential for decision-making and communicating with stakeholders.
2. Consider historical data
When analyzing current data, it's crucial to view it in the context of historical trends. A single data snapshot might not reveal the whole picture. Comparing current metrics to past performance offers valuable context and helps identify trends and changes over time. If historical data isn't available, say for a new product feature or if you're new to analyzing certain metrics, industry benchmarks can be a useful alternative. Benchmarks are available for various sectors and can be found through a simple online search, just make sure it’s from a reputable website for the most accuracy.
3. Look for things you don't expect
When you begin data analysis with specific goals in mind, there's a risk of confirmation bias, where you might only notice data supporting your preconceptions. It's important to remain open-minded, actively searching for data or trends that contradict your assumptions. Always keep an eye out for outliers - those odd data points that don't quite fit or look a bit out of the ordinary. They can either be super insightful or a sign you need to double-check your data. For instance, unexpected low website traffic following a newsletter campaign might be due to technical oversights, like sending to a test list or missing tracking parameters.
The goal of data analysis isn't just to find interesting facts (though that's a bonus!). It's about making better decisions, backed by solid data, and choosing the right tools to do this is essential. Businesses need tools that can simplify complex data sets, provide comprehensive analytics, and offer intuitive interfaces that make data analysis accessible to all skill levels. That’s where Hurree can help.
Hurree offers a versatile platform for data analysis, equipped with customization options, a variety of data visualization types, and seamless integration with your data sources. Whether you're looking to streamline your reporting process, uncover hidden trends, or make data-driven strategic decisions, Hurree can help. Get started with your free trial today and you’ll soon see how much easier data analysis becomes.
Share this
You May Also Like
These Related Stories
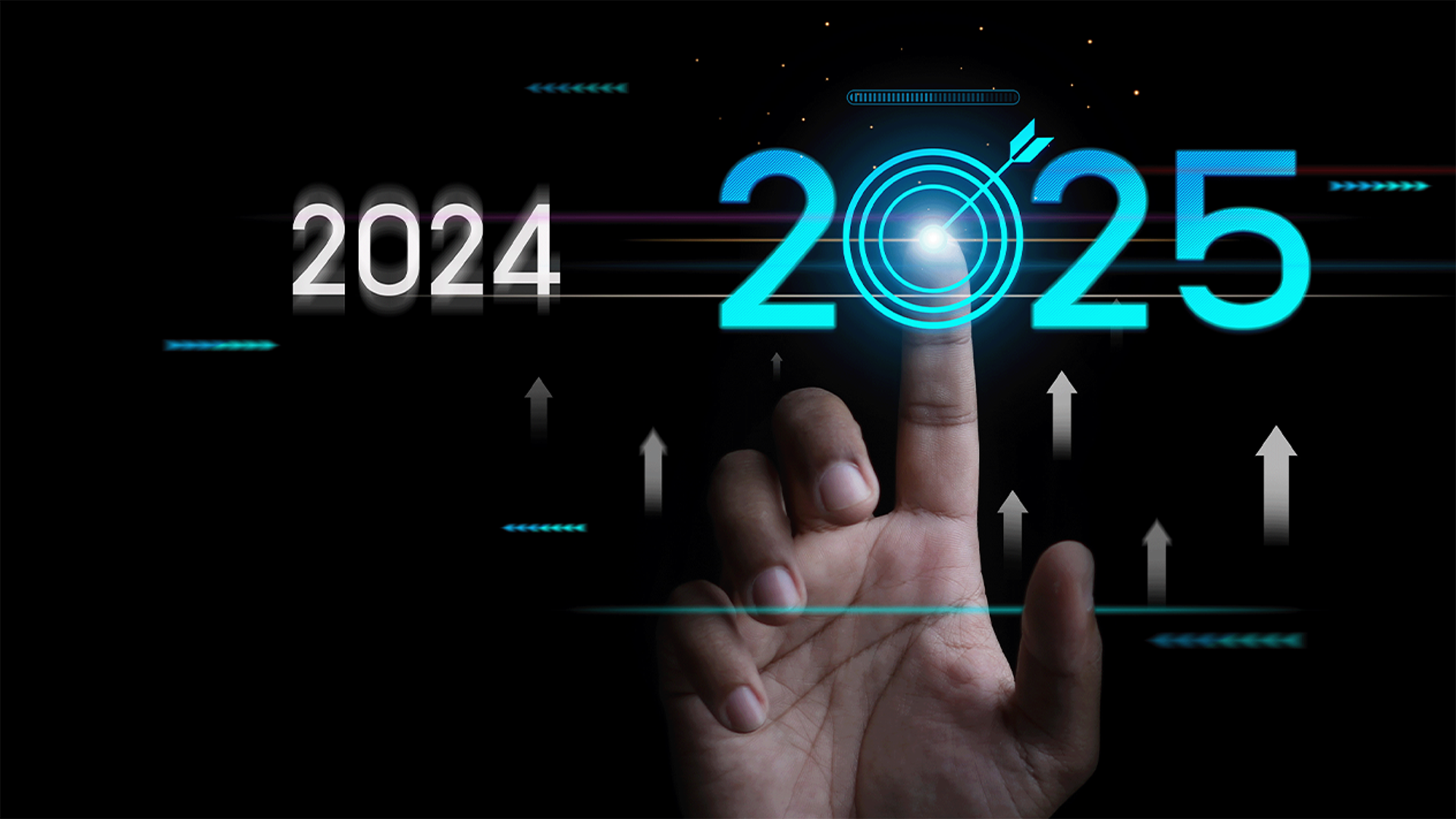
How to Use 2024 Data to Shape a Winning Strategy for 2025
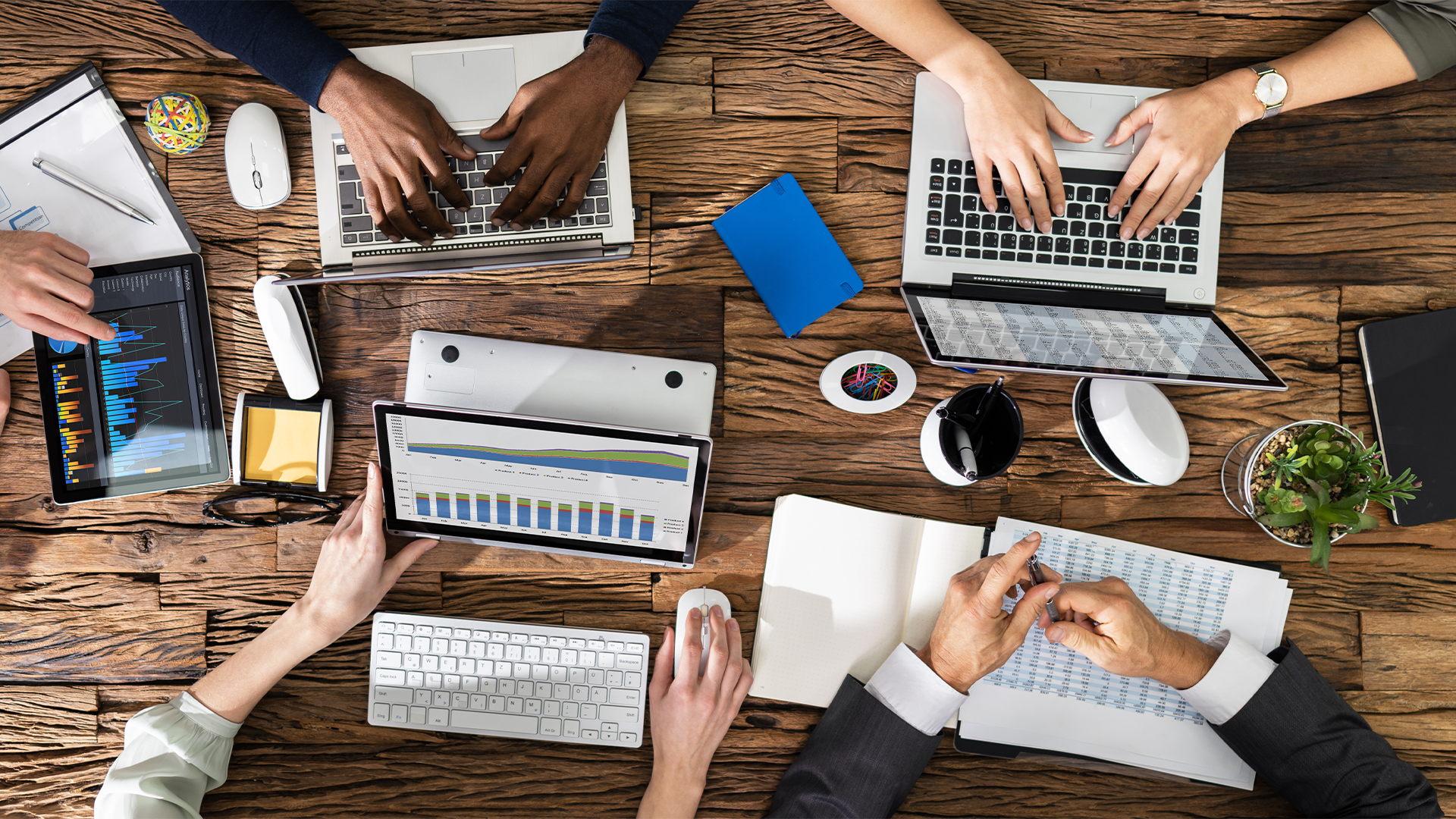
How To Be a Data-Driven Marketer: 8 Key Steps
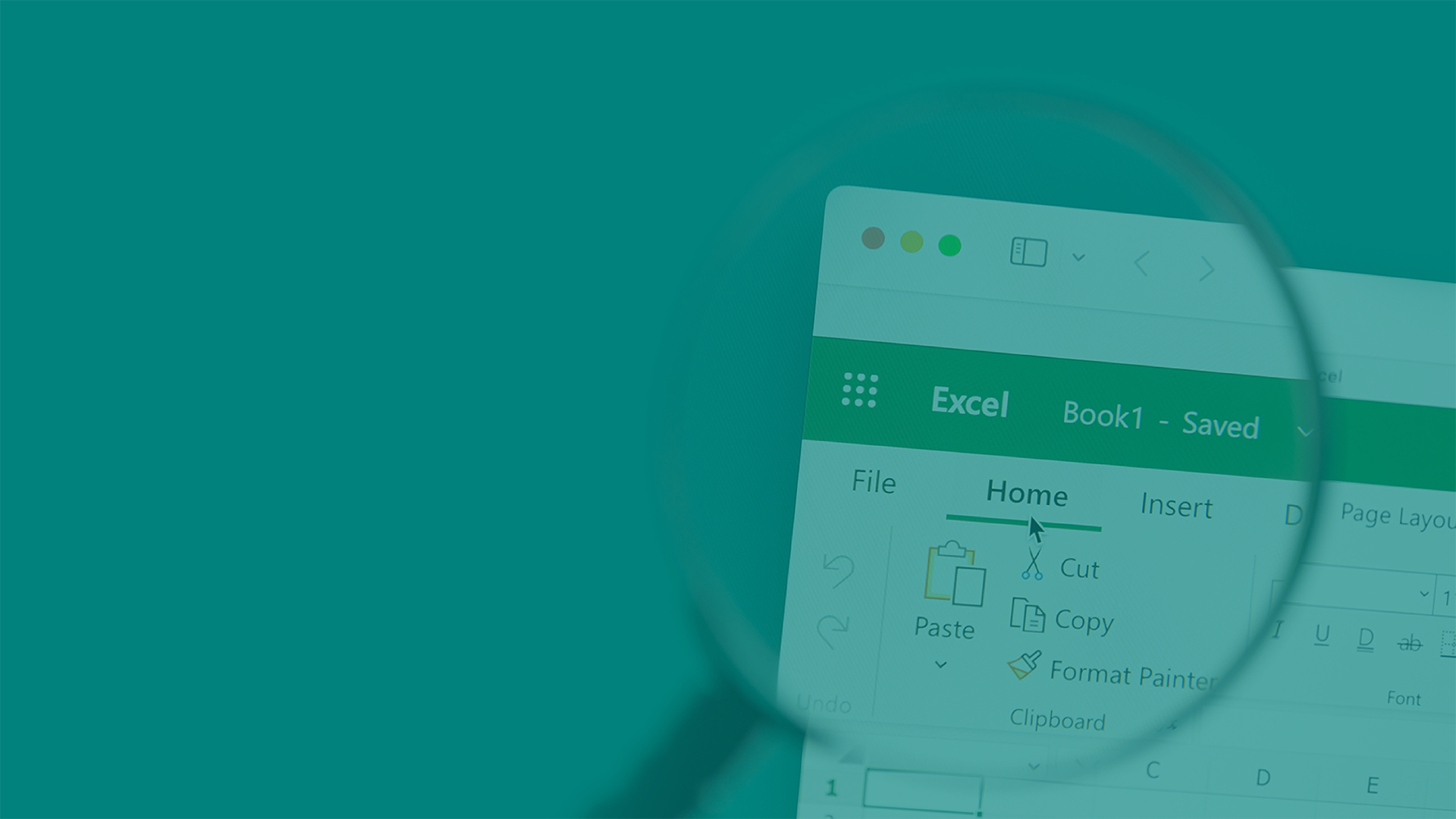