What is Data Integrity?
Do you remember the financial crisis of 2008? It had a worldwide impact that is still felt today. And do you know what caused it? Bad data (among other things). Without getting into too much detail, the 2008 crisis was fueled by bad data that overstated the value of important financial information. A lack of data integrity literally cost the world $2 trillion.
Having accurate data is vital even at a smaller scale. Gartner estimates inaccurate data costs organizations an average of $12.9 million every year. That’s why data integrity is so important.
Source: Forbes
Data Integrity Definition
In its most simplified sense, data integrity is the practice of ensuring data remains accurate, valid and consistent throughout the entire data life cycle. To understand the concept fully, you need to know that data integrity has two definitions, depending on the context in which you approach it.
Logical data integrity
First, we have logical data integrity as a process that ensures that data is kept accurate and consistent. The primary purpose of this process is to stop data from becoming compromised and, essentially, useless.
There are three basic types of logical data integrity:
- Entity integrity
When data is recorded in tables for relational databases, entity integrity ensures a primary key (id, name) for each column. No two columns have the same identity or are unnecessary (null). - Domain integrity
In data integrity, a domain is a predefined set of allowed values that data can take on when records are created, for example, the data type, the length, the range, limitations and constraints, the date format, etc. Domain integrity outlines these values and ensures that recorded data is restricted to these formats. - Referential integrity
In relational databases, two or more tables can be linked together in a ‘relationship’ because they contain related data. These relationships are created using a foreign key (the associated table) and a primary key (the primary or parent-table).
Referential integrity requires a valid primary key to be referenced in the parent table whenever a foreign key is used, thus ensuring consistency between these tables.
Physical data integrity
Second, is the product of these processes, physical data integrity as a state, i.e., a data set that is accurate and valid. Here we are concerned with storing and fetching the data to ensure it is not corrupted by events such as power outages, natural disasters, corrosion, etc. For many businesses, the introduction of cloud storage has solved the threat posed by loss of physical data integrity.
Source: Forbes
When do data integrity problems occur?
If data integrity processes are not followed, it can, as we have already mentioned, have a high cost to business, research, and anyone attempting to make decisions based on that data.
Here are some scenarios and instances where data integrity can become compromised:
- Human error - these are mistakes in data recording or maintenance, ranging in intent from accidental to malicious. Human error occurs when data activities are recorded incorrectly or wrongly deleting files, etc.
- Transfer errors - unintentional corruptions that occur during the movement of data between devices, applications or databases. Alterations, duplications of records, or undetected failed transfers can all threaten data integrity.
- Cyber threats - software bugs, hacking and viruses/malware are examples of insidious attempts to gain unauthorised access to sensitive private data.
- Compromised hardware - device or disk crashes can cause data to be corrupted or lost entirely, meaning it is no longer usable for analysis or record keeping.
- Remote working - moving from IT team controlled enterprise content management systems to off-premise remote working solutions can cause a loss of data integrity. For example, if personal laptops or USBs are used to enhance data accessibility this can lead to leaks or misplacement of sensitive data.
Source: Harvard Business Review
How can you ensure that your data is accurate and consistent when it's generated, duplicated, accessed, and moved around your enterprise at such a rapid rate?
6 pillars of data integrity
The six pillars of data quality provide a framework to ensure your data meets the highest standards. Let’s dive into these pillars and why they matter.
1. Accuracy
Accuracy is the cornerstone of data quality. It ensures that the data reflects the real-world objects or events it represents. For example, an accurate customer database will correctly capture names, addresses, and contact information. Inaccurate data can lead to miscommunications, failed campaigns, and wasted resources.
How to achieve it: Regularly validate and cross-check data against trusted sources. Employ data entry standards and error-checking tools.
2. Completeness
Completeness ensures that all required data is present and available for use. Missing information can hinder analysis and decision-making. Imagine running a marketing campaign without knowing your audience’s email addresses—a clear case of incomplete data.
How to achieve it: Identify critical data points for your business and implement checks to ensure their capture during data collection.
3. Consistency
Consistency means that data remains uniform and reliable across all systems and applications. For instance, if one system records a customer’s name as “John Doe” and another as “J. Doe,” inconsistencies can disrupt workflows and analytics.
How to achieve it: Establish and enforce data standards and synchronization processes across platforms.
4. Timeliness
Timely data is up-to-date and available when needed. Outdated or delayed information can cause businesses to miss opportunities or make decisions based on old data. For example, relying on last quarter’s sales figures to forecast next month’s inventory needs can result in overstocking or shortages.
How to achieve it: Automate data updates and monitor data freshness regularly.
5. Validity
Validity ensures that data adheres to predefined formats, rules, or constraints. Invalid data can arise from errors during entry or incompatible system integrations. For instance, entering "31st February" as a date would fail the validity test.
How to achieve it: Define and enforce validation rules, such as date formats or required fields, at the point of data entry.
6. Uniqueness
Uniqueness ensures that each record in a dataset is distinct, without duplicates. Duplicate records can lead to inflated metrics, skewed analyses, and wasted resources. For example, sending multiple marketing emails to the same person not only wastes money but also risks annoying the recipient.
How to achieve it: Implement deduplication processes and use unique identifiers for records.
Do you need data integrity for data integration?
As we discussed above, data integrity can be compromised when problems occur during the migration of data. In some forms of data integration, data is transferred and replicated between systems for communication and analytics - this is precisely when unwanted duplications or alterations occur.
Steps must be taken to avoid the corruption of data during the integration process:
- Data cleaning - the process of identifying and fixing corrupt, incorrect or inconsistent data.
- Data profiling - categorising and documenting the structure of source data to understand its content and forecast the probability for error. Data profiling can help identify integrity problems before they arise and quickly resolve them.
- Data quality rules - create custom business rules to identify which source data is accurate, essential and reliable.
Attempting to integrate data without adequate data integrity protocols can lead to wasted resources and inaccurate business intelligence, meaning you’re basing essential decisions on bad data. Not ideal.
Summing up
Poor data integrity will undermine any effort you take to be a data-driven business. It seems like common sense, yet it’s estimated that 3% of companies meet basic data quality standards.
Safeguarding data integrity can ensure:
- Quality in your products and services
- Confidence in your business intelligence and decision making
- Safety and data privacy for your customers
One way to ensure data quality is to work with tools that guarantee the integrity of your information. Hurree pulls data directly from your tech stack so you know the data will be accurate. And, unlike a lot of other tools that are built on third-party infrastructure, the Hurree platform is custom built with over 99% uptime. This means you will always have timely data that isn’t passing through numerous other platforms.
If you want to try Hurree, we offer a 7-day free trial of our professional tier, as well as a free foerever plan. You can see for yourself how a tool like Hurree is key to maintaining your data integrity.
Share this
You May Also Like
These Related Stories
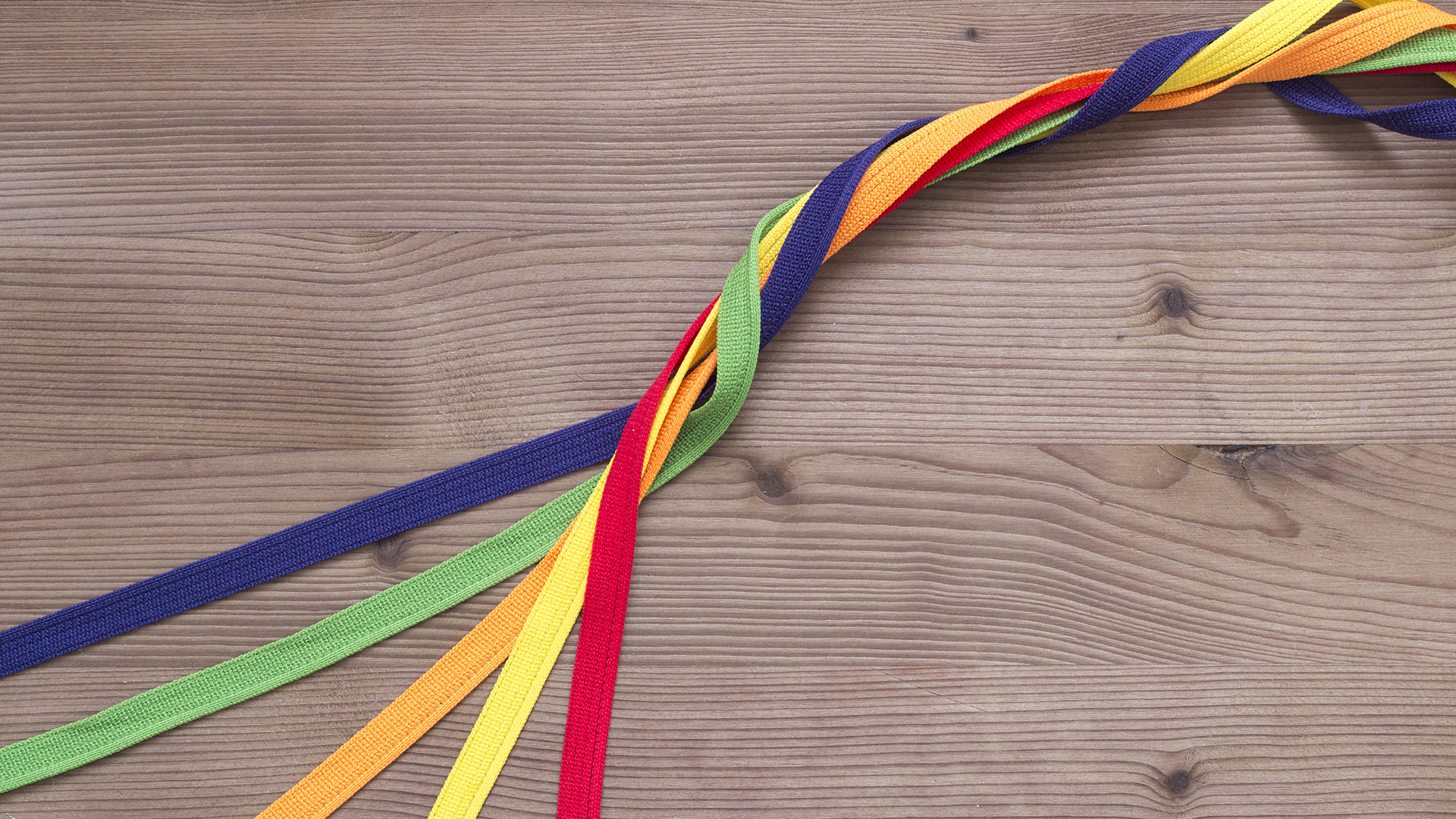
What is Data Integration in Marketing?
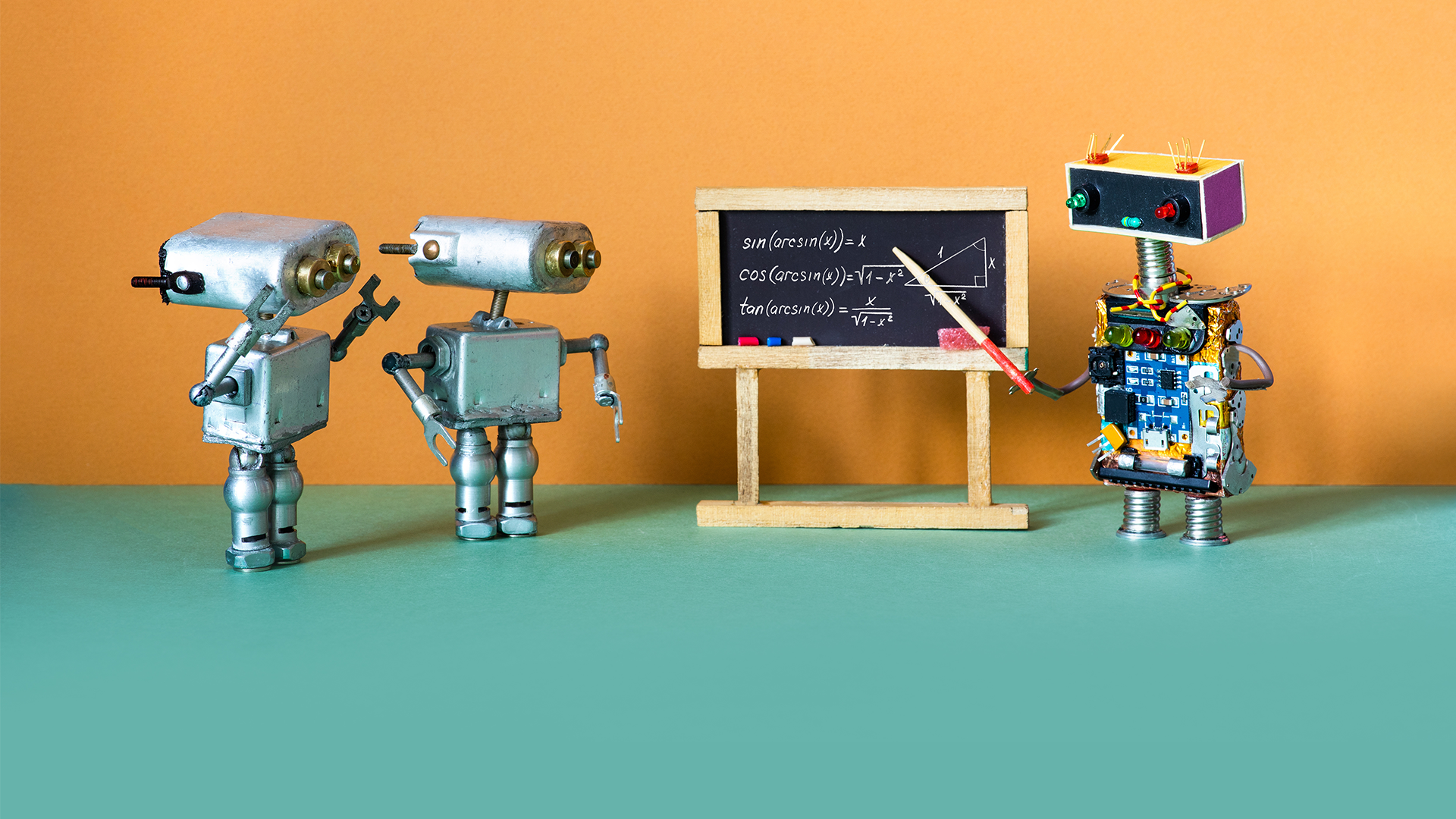
What is Data Integration? Simplified
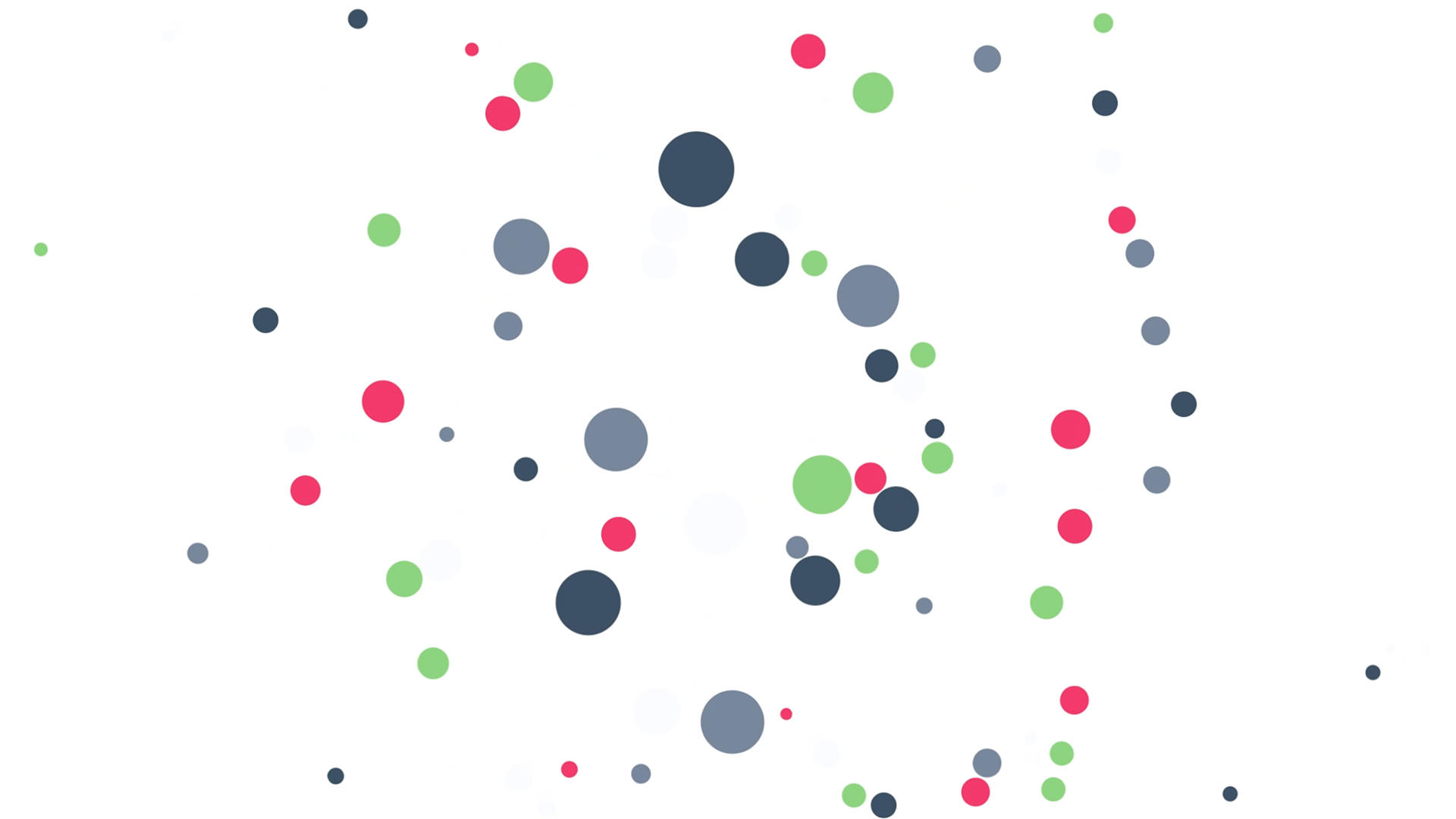